Abstract
The success of CAD techniques for the detection of abnormalities in mammographic images depends on an accurate differentiation between pectoral muscle and breast tissue. The lack of a pectoral muscle elimination step in CADs is known to degrade the performance of detection algorithms, while digitization artifacts in the background may introduce noise in the training procedure. Therefore pectoral muscle and background segmentation are important preprocessing steps for inference over these biomedical images. There is a large variation in shape, size and density of pectoral muscles in mammograms and previously to this work only shallow methods based on simple statistics and image processing filters were analysed in this tasks, yielding suboptimal and non-generalizable results. As far as the authors know, this is the first work containing experimental evaluations of Deep Learning approaches for these tasks. We show that Deep Neural Networks comprise the state-of-the-art of current breast region segmentation algorithms, achieving significantly higher results than the baselines in several objective metrics for almost all datasets and tasks evaluated in this study.
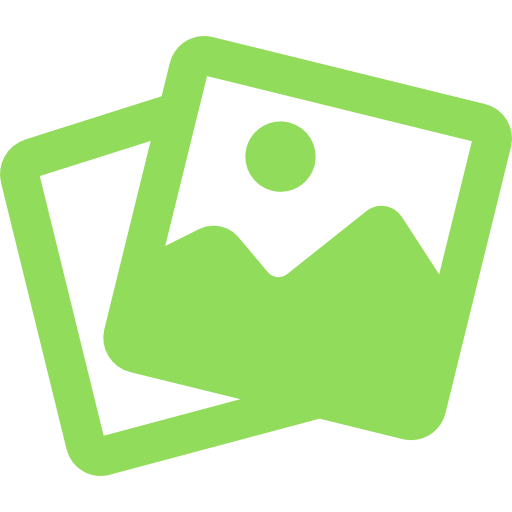